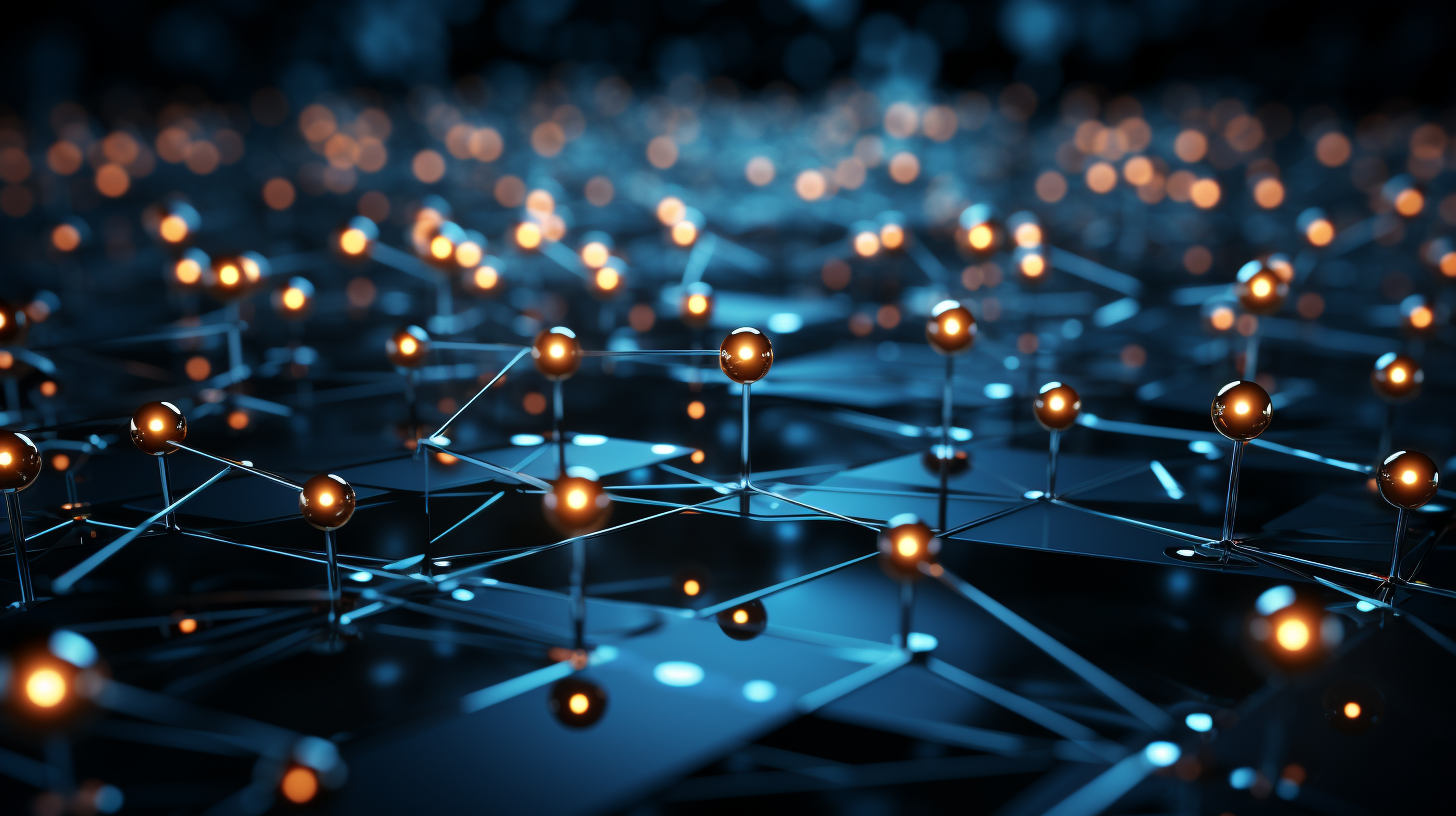
THE BLOG
Mentoring in a hybrid world - Human + Machine?
Connections - Midjourney - Antony Slumbers
Much is made of the problem hybrid, remote and distributed working causes for most companies around mentoring of new and junior staff members.
Often the response is that ‘we need to get people back in the office’’.
But is that the right response. Or the only option?
Well, it is ‘a’ response. But one that goes against the grain of how ‘normalcy’ is developing around the way we work. Put simply we cannot rely on mentor and mentee being in the same place.
Or at least not all the time.
So perhaps we need a ‘hybrid’ way of mentoring, as well as working?
Which brings us to the question ‘how can Generative AI help in the process of mentoring?'
I think, it can. A lot.
By following the outline of this proposed slide deck:
Slide 1 - Title: AI-Assisted Mentoring Program
Slide 2 - Purpose: Provide more frequent mentoring conversations through an AI assistant. Maintain human manager oversight via prompts and monthly check-ins. Goal is to enhance mentee development and growth.
Slide 3 - Mentor Preparation:
Develop a series of prompts that are open-ended, thought provoking questions tailored to mentee. These would be prepared by individual mentors and become part of a ‘Prompt Library’.
Schedule 30-60 min monthly in-person mentoring check-ins
Review AI session summary reports before check-ins
Slide 4 - AI Mentoring Process:
Manager provides prompts
AI has regular mentoring conversations guided by prompts
AI sends summary report to manager prior to their check-in
Manager has in-person check-in equipped with insights from report
Manager refines prompts based on insights and progress
Slide 5 - Sample AI Summary Report [*see below for a sample report]
Slide 6 - Action Steps:
Managers create initial prompts for mentees
Schedule first in-person kick-off mentoring session
Begin regular AI mentoring conversations
Provide feedback on experience and report format
Ultimately the aim would be to solve the Bloom Sigma Problem - as explained here:
‘Bloom's 2 sigma problem refers to an educational study by Benjamin Bloom in 1984 that found students who received one-on-one tutoring performed two standard deviations better than students in a traditional classroom lecture setting. This highlighted the significant benefits of personalised instruction and attention.
While AI mentoring has many advantages, completely replicating the effectiveness of human one-on-one tutoring is challenging.
Some key considerations:
Human tutors have deep expertise, wisdom and intuition gained from years of study and experience in their field that AI cannot fully match.
The interpersonal connection between a student and human tutor can provide additional motivation, empathy and support.
AI has some limitations in understanding full context and nuanced human communication/behaviour during complex discussions.
The datasets used to train AI mentors may lack diversity leading to biases. Humans are better able to adapt across different personalities and learning styles.
That said, AI mentoring can get closer to the 2 sigma effect through methods like:
Leveraging large datasets to train conversational ability and mentoring style.
Incorporating multi-modal feedback - verbal, visual, emotional - during discussions.
Maintaining human oversight and input to enhance AI mentor prompts over time.
Tracking detailed metrics on learning improvements to refine approach.
Utilising personalisation algorithms to tailor mentoring to individual needs.
While not a complete solution yet, AI mentoring can still dramatically expand access to the benefits of one-on-one instruction. With continual improvements, bridging Bloom's 2 sigma gap could become more feasible over time.’
So, in summary, it’s a fair amount of work to set up but I believe this could be an enormously powerful tool for companies to adopt. The development of the ‘Prompt Library’ is key. Each specialist within a company would need to tailor just the right prompts to catalyse an excellent learning experience.
Combined with regular in-person mentoring sessions we might be able to get to the best of both worlds.
Remember the 2 sigma effect. That is a goal worth pursuing.
What do you think?
-----------------------------
*Sample Report provided to each mentor prior to monthly in-person sessions:
Mentee: Sarah James
Mentor: Amanda Smith
Reporting Period: January 1 - 31
Topics Covered:
Presentation skills development - gave practice run-through of upcoming quarterly presentation and received AI feedback
Time management challenges - discussed struggles with prioritisation and setting boundaries on availability
Career advancement goals - interested in leading a cross-functional initiative this year
Key Insights:
Wants to work on voice projection and limiting verbal fillers during presentations
Has difficulty declining or delegating extra tasks from peers
Would like to gain experience managing a team for advancement
Progress Made:
Practiced presentation 3 times and improved confidence each time
Implemented 30-minute focus blocks in calendar to limit distractions
Reached out to cross-functional counterparts to discuss initiative ideas
AI Mentoring Feedback:
Appreciates consistent opportunity to practice presenting and get unbiased feedback
Found time management tips helpful but still struggles with execution
Suggest focusing next 1-1 on assembling team and pitch for leading potential initiative
Recommendations:
Provide input on body language and slide design to elevate presence
Discuss prioritisation frameworks to identify key tasks vs. "nice-to-haves"
Share insights on navigating cross-collaboration and tips for leadership
Future Real Estate Skills - Human or Machine?
Socrates meets Deep Learning - Midjourney - Antony Slumbers
What will be the highest value skills to have within the most successfull commercial real estate companies over the next 5-10 years?
1. Data Analysis & Interpretation: Transforming raw data into actionable insights, optimising investment strategies and understanding nuanced market dynamics.
2. Sustainability Expertise: Championing eco-friendly practices, resonating with a growing environmentally-conscious audience and ensuring compliance with evolving green standards.
3. Partnership Building:Cultivating strategic alliances, broadening a company's reach, resources, and adaptability in a collaborative business landscape.
4. Design Thinking: Employing a human-centric approach to innovation, creating spaces that are both functional and emotionally resonant, ensuring sustained tenant satisfaction.
5. Space Utilisation: Adapting and reimagining spaces to reflect contemporary needs, offering flexibility in design and maximising the utility of every square foot/metre.
6. Stakeholder Relationship Management: Nurturing trust and loyalty with tenants, investors, and partners, fortifying the company's reputation and ensuring long-term collaborations.
7. Marketing & Branding: Carving a distinctive brand identity, leveraging both traditional and digital platforms to capture and engage target audiences.
8. Change Management: Streamlining transitions in adopting new technologies, navigating market disruptions, and leaning into innovative business models, ensuring operational resilience and forward momentum.
9. Adaptability: Demonstrating agility in strategy and operations, preparing companies to pivot seamlessly in response to unpredictable market shifts.
10. Digital Proficiency: Integrating emerging technologies, enhancing operational efficiency, and offering a cutting-edge tenant experience, staying ahead of industry curves.
11. Strategic Forecasting: The ability to anticipate market shifts and future trends will be crucial. This skill will help companies in making informed investment decisions and staying ahead of competitors.
HOW DO WE SCORE - HUMAN OR MACHINE?
Now lets score each of these skills on their human quotient. Out of 10. The higher the score the more human input needed.
* Data Analysis & Interpretation: 6/10
While technology can gather and process vast amounts of data quickly, human intuition and expertise are still vital in interpreting the results and drawing actionable insights.
* Sustainability Expertise: 8/10
Though tech aids in monitoring and implementing sustainable systems, the strategy, innovation, and execution heavily rely on human expertise and values.
* Partnership Building: 9/10
Building relationships and forging partnerships is inherently human. While tech can facilitate communication, the essence of partnership remains a human endeavor.
* Design Thinking: 7/10
Technology provides tools and platforms, but the empathy, creativity, and problem-solving core to design thinking are deeply human.
* Space Utilisation: 7/10
Technology offers tools for modeling and visualisation, but understanding human needs and translating them into space design requires a human touch.
* Stakeholder Relationship Management: 9/10
Tools can manage data and automate communication, but genuine relationship-building, trust, and loyalty are human-centric.
* Marketing & Branding: 5/10
Digital marketing leans heavily on technology for analytics, reach, and automation, but crafting a brand story and strategy is a human endeavor.
* Change Management: 8/10
Tech can assist in the implementation phase, but understanding organisational culture, and guiding teams through transitions, is primarily a human skill.
* Adaptability: 8/10
While tech can provide data to guide shifts, the ability to be agile, resilient, and responsive to change is a human trait.
* Digital Proficiency: 4/10
The nature of this skill requires understanding and utilising technology, but humans are needed to strategise its application, integration, and innovation.
* Strategic Forecasting: 7/10
While technology and AI can analyze current data and provide predictive models, the human element is essential in interpreting these findings in context, making judgments about unquantifiable factors, and devising strategic responses. Understanding the broader socio-economic and political landscape and its potential influence on the real estate market requires nuanced human insights. Thus, while tech plays a role, the larger part of strategic forecasting remains human-driven.
SO FAR SO GOOD
The scores above were generated by a machine. And they surprised me. I thought our human skills would be considered less important than the algorithm actually did rate them. Which made me think, either it was just being polite or it is true that we underestimate just what is special, and non replicable, about being a human.
Let’s think about what humans are good at. Where do we excel?
How about:
Empathy and Emotional Intelligence: Humans have the innate ability to understand, relate to, and act upon emotions—both their own and others'. This is crucial in areas like stakeholder relationship management, design thinking, and partnership building, where understanding human needs, preferences, and emotions can lead to more effective decisions.
Contextual Understanding: While machines can process vast amounts of data, they often lack the broader socio-cultural and historical context. Humans can understand subtleties and nuances in ways that machines currently cannot.
Creativity and Innovation: The human mind's ability to think outside the box, draw from disparate fields, and come up with novel solutions is unmatched. Areas like design thinking and strategic forecasting benefit immensely from human creativity.
Ethical Judgment: When faced with decisions that have moral or ethical implications, humans use a complex interplay of cultural, social, personal, and situational factors to arrive at a decision. Machines can be programmed to follow ethical guidelines, but they don't "understand" ethics in the human sense.
Complex Problem Solving: While machines can solve well-defined problems efficiently, humans excel at navigating ambiguity, dealing with incomplete information, and addressing problems that don't have clear-cut solutions.
Intuition: Often, decisions in fields like real estate are made based on gut feelings or intuition, especially when data is lacking or inconclusive. This intuitive sense, drawn from years of experience and tacit knowledge, is challenging for machines to replicate.
Interdisciplinary Thinking: Humans can draw connections between seemingly unrelated fields or ideas, leading to innovative solutions. While machines can be trained on vast datasets, the serendipitous connections often made by humans are less predictable for machines.
Learning from Few Examples: While many machine learning models require vast amounts of data to train, humans can often learn from just a few examples, adapting quickly to new situations.
Value-based Decision Making: Humans make decisions not just based on logic or data, but also based on values, beliefs, and personal experiences.
Collaboration and Teamwork: The human ability to work in teams, leverage each other's strengths, and collaborate effectively is a complex social dynamic that is challenging to replicate in machines.
They are all good aren’t they? Powerful. But I cannot help but think most of us are not as good at these ‘human skills‘ as we should be. They may well be innate and generic to all humans but how many of us can say we have them in abundance? Not enough?
Here’s some suggestions as to how we can fix this:
Empathy and Emotional Intelligence:
Practice Active Listening: Truly pay attention to what others are saying without formulating a response in your mind.
Self-reflect: Regularly check in with your emotions and understand their origins.
Engage in Role-playing: This helps in understanding different perspectives.
Contextual Understanding:
Continuous Learning: Engage in lifelong education, reading books, articles, and attending seminars or workshops.
Travel: Experiencing different cultures can provide broader contexts and understandings.
Creativity and Innovation:
Engage in Diverse Experiences: The more varied your experiences, the more dots you have to connect.
Practice Brainstorming: Allow your mind to explore ideas without judgment.
Ethical Judgment:
Engage in Moral Philosophy: Read and debate about ethical issues.
Seek Diverse Perspectives: Engage in discussions with people from different backgrounds and belief systems.
Complex Problem Solving:
Challenge Yourself: Take on tasks or projects slightly out of your comfort zone.
Break Problems Down: Divide complex problems into smaller, more manageable tasks.
Intuition:
Trust Yourself: When faced with decisions, listen to your gut while also seeking evidence.
Reflect on Past Decisions: Understand when your intuition served you well or led you astray.
Interdisciplinary Thinking:
Study Outside Your Field: Engage in courses or readings from diverse disciplines.
Network with Professionals from Other Domains: This exposes you to different ways of thinking.
Learning from Few Examples:
Practice Rapid Learning Techniques: Such as the Feynman Technique.
Engage in Hands-on Learning: Practical experience often accelerates understanding.
Value-based Decision Making:
Define Your Core Values: Understand what matters most to you.
Regularly Re-evaluate Decisions: Ensure they align with your values.
Collaboration and Teamwork:
Participate in Team Activities: Such as sports or group projects.
Develop Communication Skills: Understanding and being understood is key to effective teamwork.
All of which feels like terribly hard work! Maybe though that is the price we are going to need to pay to stay relevant, and valued, in an AI mediated world?
And you thought your school days were over:)
Cities, AI and the Metaverse? Risks, Opportunities, Actions
The Allegory of Good and Bad Government by Ambrogio Lorenzetti, 1338 - 1339 , Siena, Italy
To answer this I’m going to take a What?, So What?, Now What? approach.
First we’ll define what we mean by artificial intelligence and the metaverse.
Then look at what can you do with AI and the Metaverse. What are they good for? And what’s happening in these sectors.
Next, the So What? What are the implications of these technologies? What are these technologies enabling? What can we do with them that was not possible before?
And finally we’ll look at what practical steps developers, asset owners or policy makers should take today?
What?
To get us going I’d like to add some context.
We are currently experiencing an extraordinary period of technological progress within the field of artificial intelligence.
You’ve probably heard of the phrase, taken from Ernest Hemingway, ‘slowly, then suddenly’. Well that perfectly sums up what has been going on since November the 30th, 2022. That was the day the AI Research company OpenAI released a ‘chat bot’ front end to their large language model GPT-3. Within just two months this new application, ChatGPT, had reached 100 million users.
Within two months! Making it the fastest growing new technology in history.
On March the 14th 2023 they released a much more powerful version, GPT-4, and then on the 23rd they announced the launch of their ‘Plugin’ service which allows integration with selected 3rd party apps and services.
One notable launch partner was the ‘Computational Intelligence’ service Wolfram Alpha. By joining forces here ChatGPT, which is a probabilistic language model, adds the ability to answer factual queries and perform advanced mathematical calculations. This extends its capabilities dramatically.
On March 21st, Bill Gates wrote the following:
‘In my lifetime, I’ve seen two demonstrations of technology that struck me as revolutionary.The first time was in 1980, when I was introduced to a graphical user interface—the forerunner of every modern operating system, including Windows.’
The second was GPT.
‘I knew I had just seen the most important advance in technology since the graphical user interface.’
He went on:
The development of AI is as fundamental as the creation of the microprocessor, the personal computer, the Internet, and the mobile phone. It will change the way people work, learn, travel, get health care, and communicate with each other.
Entire industries will reorient around it.
Businesses will distinguish themselves by how well they use it.’
Now large language models, LLMs, are not all there is within the field of AI, but the critical point is that they enable a new way of interacting with computers. Simply by using natural language, written or spoken, we will be able to instruct computers to do something. We’ll no longer need to be computer scientists to leverage the massive power of modern computational systems.
This is a pivotal moment in history. The start of an age where humans have been augmented by computers in a very profound way.
Using ChatGPT is like having an infinite number of interns at your disposal, at all times. And there will be other such systems, each one most likely finely tuned for particular use cases.
Make no mistake, this is not tech bro hyperbole. What is happening right now is genuinely a really big deal.
And our world, of cities and real estate, will be impacted in very big ways. These technologies will act like a forcing function in how people live, work and play. And of course this comes on top of the forcing function that was the pandemic.
Fortunately, all of this means we will also have better tools to help us plan, analyse and act.
But let’s deal with some descriptions first.
So what is the Metaverse?
People ascribe many meanings to it but essentially the Metaverse can be described as a persistent, shared digital universe: a vast, interconnected digital space where users can interact with one another, create and share content, participate in events, and engage in various activities.
And mostly, in those terms, it does not exist yet. The consumer version of the metaverse today is largely made up of cartoonish standalone virtual worlds, with minimal functionality, and few users. It underwent a massive hype cycle in late 2021 and early 2022 but the bubble burst and anyone who bought virtual land in any of these spaces probably wished they hadn’t.
But there is another framing of the metaverse, and that includes what are known as digital twins. These are:
‘a virtual model designed to accurately reflect a real-world object. A digital twin integrates all the data about a physical object across that object’s entire life cycle. In fact, digital twins can simulate objects in such accurate detail that they mimic every aspect of an object as if it were real.’
And these ‘objects’ can be anything from an individual, small physical item to an entire city. Digital twins can be scaled up to represent entire ‘systems’. And they can incorporate infinite data sets, representing the past, the present and anticipated futures.
And they very much do exist and will be the focus of our attention, because they are massively important in the future of cities and real estate.
Now what is AI and what is it good for?
Simply put Artificial intelligence (AI) is ‘a wide-ranging branch of computer science concerned with building smart machines capable of performing tasks that typically require human intelligence.’
But the definition is not so important. What is is an understanding of the five areas where AI excels, and these are Perception, Communication, Knowledge, Reasoning, Planning.
Perception refers to the ability of an AI system to interpret sensory data from its environment, such as images, sounds, and other signals. This typically involves tasks like object, facial and speech recognition.
Communication refers to AI's ability to understand, generate, and respond to human language. This encompasses natural language processing (NLP), natural language understanding (NLU), and natural language generation (NLG).
Knowledge refers to the ability of an AI system to acquire, store, and use information to perform tasks, answer questions, and make decisions. This encompasses knowledge representation, knowledge graphs, and the integration of structured and unstructured data.
Reasoning refers to the ability of an AI system to process information, draw inferences, and make decisions based on its knowledge. This encompasses various types of reasoning, such as deductive, inductive, and abductive reasoning, as well as common-sense reasoning.
Planning refers to the ability of an AI system to create and execute a sequence of actions to achieve specific goals or objectives. This encompasses various aspects such as goal formulation, action selection, and plan execution, as well as handling uncertainty and adapting to changes in the environment.
Think of these abilities like this: machines can understand what they are looking at, they can read and write extremely well, they can store and synthesise vast amounts of structured and unstructured data, they can apply reasoning to all that data, and they are really good at planning, particularly at scale and at speed.
There are some things us humans can beat them at across these five domains, but mostly they’ve been better than us for some time.
We’re rapidly approaching ‘don’t bring a knife to a gunfight’ territory. Understand what ‘machines’ excel at and let them get on with it.
At the same time remember what Picasso said: ‘computers are useless, they can only give you answers’. Us humans need to excel at asking the right questions.
More on that later.
That’s the What, now let’s address ‘So What’. What do these technologies enable. Can we do with them?
Just stepping back a bit, what’s the big issue we face in our cities?
I think of it like this:
With the rise of hybrid, remote and distributed working (largely as a consequence of what we learnt during the pandemic) city centre offices are much less busy than they used to be, as are retail businesses around them that used to cater to the needs of office workers.
So city centres are quieter, transportation networks are less busy, and people are moving further out in search of more space to live/work.
People want more flexibility, less commuting and more agency over their time.
So the dynamics of cities are changing.
And we need to figure out how to help our cities remain, or return to being, dynamic, thriving, enjoyable, liveable places?
So what therefore are the opportunities and risks for the real estate sector from all of this and what part can AI and the metaverse (more particularly digital twins) play.
Let’s start with risks:
The most obvious risk is around the possible, more realistically likely, decline in demand for for traditional office spaces and commercial properties, leading to increased vacancies, reduced rental income for property owners, and business taxes for the city.
And then secondly the disruption of traditional business models.
We got very used to how things worked: Everyone came into the central business district to work, five days a week, in offices on long leases. And all that footfall supported an entire ecosystem of supporting companies, as well as paid for the infrastructure to get around the city easily.
And now, mostly, this is no longer what people want.
Many employers want the old world to return, and some are having apparent success in forcing the clock back.
But the reality is that those days have past.
The pre pandemic world is not coming back. Employees want different things, and are pretty determined to get them.
The most talented employees have a lot of optionality; they are in demand and so can demand ‘a better way’.
There really is no doubt that companies that are slow to adapt to these changes will face competitive disadvantages and potential obsolescence.
And talking of obsolesce, that will be the fate of a great deal of real estate over the coming years.
The real risk to anyone involved in real estate or managing and running cities is NOT leaning in to these changes, or underestimating them.
We’ve had two years of denial from many leaders; everyone will be back soon, they’ll be back after the summer holidays, come the new year etc etc…
And at every turn they’ve been disappointed.
As Robert Frost wrote ‘the only way round, is through’.
Accept, and embrace, then act.
For opportunities they are aplenty.
Looking at those directly related to AI & Digital Twins I’d like to concentrate on three:
Enhanced property management
AI-driven urban planning and development
Adaptive reuse of existing spaces
We need to dive deep into these, because by doing so we’ll cover all the key ways these technologies can help us achieve our goals. Thought processes, workflows, software and hardware used in these examples will be transferrable to many other situations. The AI & Digital Twin opportunity space is deep, and wide.
Enhanced property management.
How can companies create office spaces that are not only more efficient and sustainable, but also more appealing and enjoyable for employees. That encourage in-person collaboration, enhance employee satisfaction, and ultimately, make offices a desirable destination for people to work and interact.
How can AI be leveraged to help achieve this:
Workspace personalisation:
AI can analyse individual preferences, work habits, and schedules to tailor office spaces according to each employee's needs. This can include adjusting lighting, temperature, and desk arrangements, providing a more comfortable and enjoyable work experience.
Smart meeting scheduling:
AI can optimise meeting schedules based on participants' availability, preferred meeting times, and locations, reducing conflicts and improving the overall meeting experience. Meetings are a constant bugbear - AI can make them better.
Enhanced collaboration tools:
AI can be integrated with collaboration tools to streamline communication, facilitate idea sharing, and support decision-making. Features such as real-time transcription, language translation, and intelligent content summarisation can help create a more inclusive and engaging work environment, fostering collaboration and innovation.
Health and well-being:
AI can be used to monitor and analyse environmental factors, such as air quality, noise levels, and natural light, and make adjustments to improve the overall well-being of employees. Additionally, AI-powered wellness programs can provide personalised recommendations for breaks, exercises, and mental health support, contributing to a healthier and more balanced work-life experience.
Efficient resource management:
AI can optimise the utilisation of office resources, such as meeting rooms, workstations, and shared amenities, ensuring that these spaces are available when needed and used efficiently. This can reduce overcrowding, improve overall employee satisfaction, and create a more organised work environment.
Dynamic workplace layouts:
AI can analyse data on how employees interact with their physical environment, identifying patterns and trends that can inform the design of adaptive, flexible workplace layouts. This can help create office spaces that foster collaboration, creativity, and productivity while still providing areas for privacy and focused work when needed.
Enhanced security and access control:
AI-powered security systems can provide more effective and non-intrusive access control, using technologies like facial recognition, behaviour analysis, or biometrics. This can help create a secure environment while minimising disruptions and inconvenience for employees.
Real-time feedback and analytics:
AI can collect and analyse real-time feedback from employees regarding their experiences in the office. This information can be used to identify areas for improvement, monitor the impact of implemented changes, and continuously refine the work environment to cater to the evolving needs and preferences of employees.
Together, these AI-driven use cases converge to create a truly exceptional user experience for those in the office, designed to help people be as happy, healthy, and productive as possible.
They embody the essence of "space-as-a-service" initiatives, where the goal is to understand people's "jobs to be done" and provide them with spaces and services that optimise their time in the office.
In this high-tech, high-touch world, quantitative data is harnessed to craft a qualitatively superior experience.
Next, AI-driven urban planning and development
AI-driven urban planning and development can greatly contribute to the creation of more liveable and sustainable urban spaces by leveraging data analysis and predictive modelling. Here are some key ways:
Site selection and land use optimisation:
AI can analyse various factors, such as land use, Zoning regulations, environmental conditions, and infrastructure availability, to identify optimal locations for new developments. This can help developers maximise the value of their investments while minimising the impact on the environment and existing communities.
Demand forecasting:
AI can process vast amounts of historical data and current trends to predict future demand for different types of properties, such as residential, commercial, and mixed-use developments. This allows developers and city planners to make data-driven decisions about the scale and type of projects needed to meet future demand and avoid oversupply.
Transportation and infrastructure planning:
AI can analyse traffic patterns, public transportation usage, and pedestrian behaviour to optimise transportation networks and infrastructure. This can lead to reduced congestion, improved accessibility, and more efficient use of resources.
Environmental sustainability:
AI can help identify opportunities for integrating green spaces, renewable energy sources, and energy-efficient technologies into urban developments. This can contribute to more sustainable, eco-friendly cities that prioritise the well-being of both residents and the environment.
Community engagement and public feedback:
AI can be used to collect and analyse public feedback on proposed developments and urban planning initiatives. This enables city planners and developers to better understand community needs, preferences, and concerns, and incorporate them into the planning process. By incorporating public input, urban spaces can be designed to be more inclusive and responsive to the needs of the community.
Socio-economic impact analysis:
AI can analyse the potential socio-economic impacts of proposed developments, including factors such as job creation, housing affordability, and access to essential services. This can help ensure that new developments contribute positively to the overall quality of life in urban areas and promote equitable growth. Very much the S in ESG.
Resilience and adaptation planning:
AI can be used to evaluate the potential risks and vulnerabilities of urban areas to climate change, natural disasters, and other hazards. By identifying these risks, city planners and developers can incorporate resilience measures and adaptive strategies into urban designs, ensuring the long-term sustainability and safety of urban environments.
Simulation and scenario analysis:
AI can be used to create realistic simulations and evaluate various development scenarios, helping planners and developers to visualise the potential outcomes of different planning decisions. This can lead to more informed decision-making and better alignment with long-term goals and objectives.
In essence, the fusion of AI-driven urban planning and development heralds a new era of informed, data-driven, and responsive approaches to creating liveable and sustainable urban spaces.
By embracing AI, city planners, developers, and other stakeholders can work in concert to construct cities that are better equipped to meet the diverse and ever-evolving needs of their inhabitants.
And now, Adaptive reuse of existing spaces
AI and Digital Twins can be used together in the adaptive reuse of existing spaces by combining data analysis, simulation, and optimisation techniques. This synergy can help real estate developers identify the most suitable strategies for repurposing under-utilised properties and maximising their value.
Let’s have a detailed look at how AI and Digital Twins can work together in this context:
Data collection and analysis:
AI can be used to gather and analyse vast amounts of data on building conditions, local market trends, community needs, and regulatory constraints. This information can help identify under-utilised properties with the greatest potential for adaptive reuse and provide insights into the most suitable new uses for those spaces.
Building performance assessment:
Digital Twins, which are virtual representations of physical assets, can be used to simulate and evaluate the existing performance of a building. This includes factors such as energy efficiency, structural integrity, and indoor environmental quality. By analysing this data, developers can identify the necessary improvements and modifications required for adaptive reuse projects.
Design optimisation:
AI algorithms can be used to generate and evaluate multiple design options for the adaptive reuse of a building, taking into account factors such as cost, functionality, aesthetics, and sustainability. Digital Twins can be used to simulate the impact of these design options on the building's performance, helping developers choose the most optimal solution.
Stakeholder engagement:
AI and Digital Twins can facilitate more effective communication with stakeholders, such as local authorities, investors, and community members, by providing data-driven insights and realistic visualisations of proposed adaptive reuse projects. This can help build consensus and support for the projects, ensuring that they meet the needs and expectations of all relevant parties.
Construction planning and management:
AI can be used to optimise construction schedules, resource allocation, and logistics, ensuring that adaptive reuse projects are completed efficiently and cost-effectively. Digital Twins can be used to monitor the progress of construction and detect potential issues early, allowing for timely adjustments and minimising the risk of delays or cost overruns.
Post-occupancy evaluation and optimisation:
After the completion of an adaptive reuse project, AI and Digital Twins can be used to monitor and assess the performance of the repurposed space. This can include factors such as energy consumption, indoor environmental quality, and user satisfaction. By continuously collecting and analysing this data, developers can identify opportunities for further optimisation and improvement, ensuring that the space remains functional, efficient, and appealing to users.
In summary, the combination of AI and Digital Twins in the adaptive reuse of existing spaces can help real estate developers make more informed decisions, optimise designs, and improve project outcomes. By repurposing under-utilised properties into new, innovative spaces, developers can contribute to the vibrancy and diversity of urban environments while making the most of existing real estate assets.
From these three use case examples you can see that they are leveraging the core capabilities of AI, the Perception, Communication, Knowledge, Reasoning and Planning we discussed earlier.
And via a digital twin creating a virtual representation of the actualité of a physical space.
Combining these is enormously powerful.
Not only does it enable you to really understand how places and spaces are being used, but it also enables you to test out various optimisation strategies in a virtual environment prior to rolling them out ‘in real life’. Real life is very expensive to tinker with - virtual life is not. Far better to test run scenarios first.
So that’s the What and the So What around AI, the Metaverse and its critical component in our case, Digital Twins.
Now let’s look at Now What? What is it that you, as developers, asset owners or policy makers, need to do from today onwards to bring all of this together?
In a nutshell you need to consider a strategic approach that focuses on data, skills, technology, and product/service selections.
So here are six essential actions to take:
Establish clear goals and priorities:
Identify your key objectives and desired outcomes. This may include improving efficiency, enhancing sustainability, or promoting equitable growth. It is essential you are clear about what you are trying to achieve. You have to have a North Star to aim at, a definite purpose.
Invest in data infrastructure:
Robust data infrastructure is essential for harnessing the full potential of AI and Digital Twins. Develop a strategy for collecting, storing, and managing data from various sources, ensuring data privacy and security.
This may involve collaborating with partners, such as technology providers, data analytics firms, and research institutions.
Either way, until you have your data sorted out you cannot move forward. This is non negotiable. Without a solid data infrastructure you will fail. Not might, will.
Develop a skilled workforce:
Invest in training and talent development to ensure your team has the necessary skills to understand, deploy, and manage AI and Digital Twin technologies. This may involve upskilling existing employees or recruiting new talent with expertise in data science, machine learning, and digital simulation.
However, skills in this area are very much a moveable feast; a constantly evolving menu to pick and choose from. So whilst it is important that your core teams understand the fundamentals around these technologies, in particular what they are good at, and not good at, it is most likely you will need to build a network and ecosystem of partners whom you can call on for specialist, and ongoing assistance.
Have someone on your team who is constantly looking at, and researching, new tools and techniques. And have them regularly appraise the C Suite as to the changing landscape. Frankly it is almost impossible to keep up fully, but you mustn’t slip far behind. It’s a relative game; always try to be one step ahead of your peers.
Identify use cases and pilot projects:
Start by identifying specific use cases and projects where AI and Digital Twins can deliver tangible benefits. Focus on pilot projects that can demonstrate early successes, generate insights, and build momentum for broader adoption. Iterate from the use cases we discussed earlier, as those definitely work. Again, concentrate on what the technology is good at.
Evaluate technology and vendor options:
Research and assess various technology platforms, tools, and vendors that can support your AI and Digital Twin initiatives. Engage in conversations with vendors and industry experts to gain a comprehensive understanding of the available solutions and their potential impact on your organisation. This fits neatly with the need to develop a skilled workforce. Everybody should be a scout for new technologies and new vendors.
And the last essential action is to embrace a culture of innovation:
Encourage a culture of experimentation and continuous learning, allowing your organisation to adapt and thrive in an evolving technological landscape.
Be open to new ideas and approaches, and maintain a forward-looking perspective to stay ahead of industry trends and emerging opportunities.
Most companies say they do this, but in reality most either do not or do not do it well.
Given the speed of technological change, around AI in particular, and the broad and deep impact it is going to have on all of us in the next few years, it really is imperative to double down on becoming an instinctively innovative company.
We have all got a little tired of people crying wolf, or screaming ‘it’s different this time’ - but that does not mean there never is a wolf or actually, it is different this time.
Remember what Bill Gates said - what is happening in AI today is only the second utterly transformational technology he has known.
And that technology is being integrated into everything we have talked about today. Innovation is something we want to impose on ourselves, rather than have it imposed on us. Act accordingly.
So What, So What, Now What? Let’s Recap.
We are living at a time of massive technological change. After decades of promise but mostly underachievement, we have hit a confluence of data availability, compute power and algorithmic sophistication that has catalysed the creation of Large Language Models, AI programs that by learning the patterns and connections between words and phrases enable us to use natural language to interrogate ‘all the worlds information’.
Machines can now understand and create images and text, they can analyse and synthesise massive corpuses of data and information, and they can simulate the availability of infinite interns working for each of us personally, on-demand and at warp speed. In essence we have all been given access to hitherto unavailable computational superpowers.
And maybe these have come just in time, because the after effects of a global pandemic have reset how we all think about work, where we do it and what we want from it. In turn our cities are being repurposed; how we use them, and what we want from them has been fundamentally upended. With great consequences. They need redesigning.
So we need to embrace these new technologies to help us in this redesigning process. Amongst other things we can use them to enhance property management, drive new forms of urban planning and development and learn how to adaptively reuse existing spaces that are no longer fit for purpose.
Our mission, and we need to think of it like this, is how can we leverage technology to create, and then curate, a better built environment for all. That is sustainable, that is human-centric and that embraces all our humanity. It is doable, but the risk of failure is high. The problems are wicked.
We’ll need all the technology we can lay our hands on, but none of it will work unless we up our games as humans.
We need to think, feel and do like we’ve never done before.
We need to unearth our own ‘superpowers’!
Good luck.
Generative AI for Real Estate People - Conclusion/Summary
Summit - One Vanderbilt - New York - Antony Slumbers
Over two long articles (Part 1 here) and (Part 2 here) we’ve looked at how Generative AI could impact on, and be utilised by, Real Estate People. And I specifically say people because in many ways it is going to be easier, and quicker, for individuals to adopt these new tools than it is real estate companies. They will also have very meaningful personal impacts on a lot of ‘People’ so it is vital that individuals push ahead with learning about these new technologies, rather than wait for their companies to do so, as they need to be very cognisant of their own ‘utility’.
So what have we concluded?
First is that we have each been given new ‘superpowers’ - Generative AI provides us all with an infinite army of virtual interns, at our beck and call, day or night, anywhere. Our job is to leverage them.
AI has been around a long time. The term itself was coined in 1955, but the date that matters for us is November the 30th 2022, which was the day OpenAI launched their new product ChatGPT.
Within two months it had a 100 million users and a month or two later increased dramatically in power.
Critically, GPT-4, which is the ‘Foundation Model’ (known as a Large Language Model, or LLM) underlying the ‘Chat’ front end is now multi model, which means it can deal with text and images. And the version you are using today is the worst one you ever will. It will have a hundred times greater computational power in 10 years time, 8000 in 20 years and a million in 30 years. Today one can assume it has read everything. Certainly everything in English. Now imagine it has also seen everything, and heard everything. What might its capabilities be then?
Today it is excellent at text generation and understanding, plus generating images, music or sounds. As well as having very powerful predictive modelling capabilities. It is also an extraordinary teacher - personalising how you wish to be educated in anything is a joy, that in time hundreds of millions of people will benefit from. ‘Teach the world’ might well be its ultimate purpose.
But in a business capacity it is, out of the box, not very usable by companies, because of security and privacy issues. What you enter information into the public version it becomes just that ….. public. So there is a rapidly developing ecosystem of suppliers building new tools, around two main purposes. Either being able to converse with the LLM around private matters, or integrating one’s own, proprietary data into the system. Both at a company and industry level. There is a lot of customisation going on. A lot of work to create domain specific applications.
Broadly speaking the five areas that can make the most of Generative AI are marketing and sales, customer service, operations, software development and research & development.
In many of these the fundamental strength is making tacit knowledge explicit. Learning from the best in order to teach the rest.
Broken down, utility can be found in areas like the following. Within the legal world it might be around document automation, legal research, dispute resolution and predictions, risk assessment, personalising client interaction, IP creation and protection, case strategy development, legal analytics (such as trends in case law), e-discovery, training & education, and legal coding.
Within commercial real estate it could impact on design, asset performance prediction, location analytics, leasing strategy, property management, tenant screening, space utilisation, market demand forecasting, energy efficiency, valuation and marketing.
In other words, across many of the day to day tasks within any business.
Which is why, like electricity and the internet, it is widely considered a GPT - a General Purpose Technology.
And those get in to everything.
But, and this is an important caveat, the ultimate benefit of these technologies will only surface when the entire operating model of a business is designed around them, with each part talking to each other and exposing and consuming data from across the enterprise.
Of course none of this will be easy, especially as this is a fast developing area of technology. But whoever does crack it will be mighty competitive.
You need to be prepared though, as an individual and as a company.
At a general level the most important thing is to have a culture of innovation, followed by the other absolute imperative, which is having your data in order. Without both of these you’re not going far.
You also need to be continuously learning, and to have a process by which you can measure the effectiveness of this learning. You need, as in a software company, to ‘Build, Measure, Learn’. And to do this up and down the organisation. Everyone needs to be trained to use these tools, to contemplate use cases and to be thinking about what needs to happen for anything to happen.
If you are in the C Suite you need to enable all off this by making available the resources, the investment and perhaps most importantly of all the ‘air cover’ that will make it possible.
Being more specific you will need, internally or through partners, access to advanced AI literacy, data and programming skills, people with a creative mindset and design thinking skills. And you should look out for, and encourage, those with great domain expertise AND great communication skills. Technical people who can understand and be understood by non technical people. And vice versa.
And above all else you need a workforce with strong abstract and critical thinking skills. In an environment when you never know what is true or real, you must have the ability to discern which is which.
As it is ‘the machines’ that know everything the specialist becomes less imperative. People with generalist skills, and an ability to understand connections and see the big picture will be at a premium.
There are, of course, many risks, concerns and ethical implications with Generative AI.
The most talked about is their ability to hallucinate, to make things up, to sound entirely plausible whilst being absolutely wrong. You need to remember that an LLM is not a database, it's a prediction engine. It is trying to predict what a good answer to the question being asked would look like.
So it is very likely that you need to keep a human in the loop. In fact, within more complicated businesses you should probably develop your own taxonomy as to when the AI can work autonomously, and when not.
You also need to look out for, and plan around misinformation, deep fakes, IP infringement, bias, unfairness, privacy and data protection, consent & permission, security & malicious use, psychological & social impact, accountability & attribution. None of which is meant to put you off, rather to emphasise that there are serious matters that need to be dealt with seriously.
Over time it is a certainty that legislation and accepted norms are going to change. This is a moveable feast, so be agile.
How quickly is all of this going to come to pass? What is the timetable to adoption?
McKinsey recently issued a new report looking at the impact of Generative AI on business. Compared to their last major review, in 2017, they say
‘the total percentage of hours that could theoretically be automated by integrating technologies that exist today has increased from about 50 percent to 60–70 percent.’
And go on to make three other very pertinent points:
First, that based on historical findings, technologies take eight to 27 years from commercial availability to reach a plateau in adoption. It is often slower than you think, especially in large companies, because…. Well they are large, complicated, unwieldy beasts that have been fined tuned to operate in a particular manner. Changing that is hard.
Second that automation adoption is likely to be faster in developed economies, where higher wages will make it economically feasible sooner.
And third that technologies could be adopted much more rapidly in an individual organisation.
Put it all together and the implications are that we are set for rapid change!
So what will the impact be on professionals & jobs?
It might be painful.
Well known computer scientist Pedro Domingos recently tweeted this:
‘AI is the revenge of the working class: now it's the middle class's turn to fear for their jobs.’
And referring back to that McKinsey report he may have a point. Because they write that:
‘Generative AI is likely to have the biggest impact on knowledge work, particularly activities involving decision making and collaboration, which previously had the lowest potential for automation.’
Because …
‘its capabilities are fundamentally engineered to do cognitive tasks’.
And that the …
‘potential to automate the application of expertise jumped 34 percentage points’
Indeed …
‘many of the work activities that involve communication, supervision, documentation, and interacting with people in general have the potential to be automated by generative AI’
It is too big a topic for here but two thoughts are worth bearing in mind:
First, when AI reduces the cost of writing software down to almost zero we might well see a vast increase in new software that enables us rapidly and widely to create many many new and better, faster, cheaper products and services. When the cost of intelligence trends towards zero we ‘might’ unleash all manner of new opportunities.
And secondly, with lawyers in mind, when AI enables everyone to sue everyone, everywhere, all the time, the sheer scale of new work might keep them all gainfully employed for a long time to come.
Do you remember the ‘Jevons Paradox’ which proposes that ‘increases in energy efficiency may lead to an overall increase in energy consumption, rather than a decrease, as people use the efficiency savings to consume more.’
Historically new technologies have killed off jobs but what they enable has led to many more, new ones. This is not a Law of Physics, and often the gap between the old and new jobs leads to painful dislocation, but the Jevons Paradox ‘feels’ like being appropriate in a Generative AI world.
Even if it is though, who benefits will be down to us, to society, to decide. There is definitely no Law that states technological process is a naturally common good. Daron Acemoglu and Simon Johnson, in their recent book ‘Power & Progress’, make the point that new technology does not necessarily lead to higher living standards, but when it does it is a consequence of societal norms and beliefs that ‘will’ that to be the case.
Either way, general purpose technologies lead to great change. We’re just not sure where, and how.
And then lastly what impact will Generative AI have on real estate and cities?
For this I am going to refer you to my 5 in the series set of articles about ‘Four Great Real Estate Challenges’, and my long article on ‘Cities, AI and the Metaverse? Risks, Opportunities, Actions’.
Generative AI will have an enormous impact on real estate / cities as one, and a very important one, of the forces currently at play. Between the two articles I cover most of what you need to know in some depth.
So that’s it. Generative AI for Real Estate People - hold on to your hats because this is going to be one hell of a ride.
Generative AI for Real Estate People, in 10 Steps - Part 2
Summit - One Vanderbilt - NYC - Antony Slumbers
Part 2 - 6-10
6. Preparedness & Skills
Let’s start at the organisational level.
It’s safe to say that an organisation or company that does not have a culture of innovation is going to struggle getting the most out of Generative AI. It is essential that there is a willingness to adopt new technologies, a preference against maintaining the status quo, and a desire to push for new opportunities with an acceptance of possible failure. You don’t need to go all in on ‘move fast and break things’ but you do need to be comfortable with change.
After that, the most important input will come from having your data in order. The more rich and granular, digital, data you have the better. So much of the power of Generative AI comes from its ability to ingest very large quantitates of data and make sense of it. Clearly if you don’t have much that limits what is possible. That said, Generative AI works very well with unstructured data, such as emails, notes, correspondence, documentation, so is more forgiving than other technologies. And of course, if you are working with a foundational model like ChatGPT you are already working with ‘all the worlds data’, so there is much you can still do. You just won’t be able to tailor outputs to your own precise requirements.
As has been repeated for decades, real estate needs more and better data. These new technologies just re-emphasise the point.
A bias towards continuous learning should already be an organisational imperative but if it isn’t you need to start now. The speed at which these technologies are developing necessitates ongoing curiosity, retraining and re-skilling. You will always be behind the curve, but you must not see it move out of sight. So learning from all stakeholders, internal and external, is vital.
The last essential organisational level trait is to have in place systems to regularly evaluate the effectiveness of any initiatives and to rapidly course correct as necessary. Think ‘Build, Measure, Learn’ at all times.
At the individual level there are many steps to take to be prepared for a Generative AI world. Normally one would think these will differ dependant on whether you are an entry, mid or C Suite level person, but in this case I think one should assume the best ideas, tactics and strategy ‘might’ come from anywhere. So, whilst it is clearly the job of the C Suite to lead they need to be very fluid as to where they take their insights from.
With that in mind, here are some steps each of us should take:
Your organisation or company should be offering you training in these new technologies, but if they are not, do it yourself. There is a great deal available for free online and, offering more structure and help, many paid for courses. Knowledge of new tools is a serious competitive advantage that will repay just about any investment. Whoever you are.
Use the tools. Play around with the free versions of ChatGPT or MidJourney or any number of other offerings. Just getting a feel of what is possible makes a big difference.
Note down ideas about possible use cases. This is especially useful if done by front line employees who often see the actualité of how a company operates, and what it looks like to customers, in a way that more senior managers don’t.
Normally one would task mid level managers with identifying areas where AI can create the most value and developing a roadmap for implementation but frankly they might not be in the best place to do so. Let ideas flourish from top to bottom.
They are though most likely to have to handle the almost inevitable change management programs that these new technologies are going to necessitate. More on this later, but again this might need a more collaborative approach than is often the case.
C-Suite executives will be responsible for signing off on key strategic goals, roadmaps and overall vision, but before they do that their real value will come from enabling the above and making available the investment and resources to do the job well. This is not an area for top down diktats but it sure does need top down air cover, commitment and drive.
That covers general preparedness but what specific skills do companies require in their employees to effectively harness and leverage the capabilities of Generative AI?
First off the more people who understand the fundamentals of Generative AI the better. They don’t need to be practitioners but they do need to understand what it is, broadly speaking how it works, its strengths and weaknesses, and how to effectively utilise its capabilities.
You will need access to people with skills in data analysis and manipulation: proficiency in data analysis and manipulation is essential for working with Generative AI models. So work out where you will find people who have skills in preprocessing and cleaning data, as well as the ability to extract meaningful insights from datasets used to train Generative AI models.
Likewise with programming and software development: strong programming skills are necessary to implement and deploy Generative AI models. So someone needs to be proficient in programming languages commonly used in AI, such as Python, and have experience with relevant libraries and frameworks like TensorFlow or PyTorch.
Employees with a creative mindset and design thinking abilities can leverage Generative AI to produce innovative solutions, designs, or content that adds value to the business. So seek them out!
It goes without saying that domain expertise is super valuable when working with Generative AI. Especially if working on fine tuned systems for specific industries. But what you really want is people with domain expertise who can communicate extremely well with AI technologists. Being able to clearly explain requirements, inputs and desired outputs is a super skill. Look hard for these people.
Everyone needs to have a notion of ethical behaviour. More on this later.
Likewise everyone needs to develop their abilities with regard to abstract and critical thinking. Employees with strong critical thinking and problem-solving skills can effectively identify opportunities where Generative AI can add value. They can analyse complex challenges, define clear problem statements, and apply Generative AI techniques to develop innovative solutions. These skills can be taught and really are going to become critical skills in companies leveraging increasingly powerful technologies.
So we all need to be prepared and up-skill ourselves appropriately. Hopefully the above give you some ideas to where to start.
7. Risks, Concerns and Ethical Implications
Let’s start with the most common ‘risk’ mentioned around Generative AI, and that is its tendency to ‘hallucinate’.
Hallucinations refer to instances where AI models generate content that may sound plausible but is factually incorrect or completely fabricated. This can lead to misinformation and further exacerbate the challenges of trust, reliability, and authenticity in the digital landscape.
Hallucinations in Generative AI can occur due to various reasons, including limitations in the training data, biases in the learning process, or the inherent complexity of modelling real-world phenomena accurately. When relying on AI-generated content without proper verification, there is a risk of spreading false information or reinforcing misleading narratives.
Whilst the degree of hallucinating has reduced (very much so between GPT-3 and GPT-4) it is a reality that is unlikely to fully go away. In much the same way as humans are not inherently trustworthy, neither are machines.
So we need to be very careful about where we use Generative AI and when we need to maintain a ‘human in the loop’. Roughly speaking one should not use human less systems where the implications of being wrong are great or severe. Such as in medical diagnosis. If giving a definitive answer is vital then these are not the systems to rely on. One could easily be opening up levels of risk in one part of an organisation by using them in another.
It’s worth thinking about creating a taxonomy of when to have a human in the loop and when not to. This will develop over time, as new techniques and tools are developed to mitigate hallucinations but the principal remains valid. Risk management of Generative AI is an important area.
A rather hilarious recent example of the need for taking care happened with a lawyer in the US who used ChatGPT to help in putting together a defence for a client in a court case. The AI duly produced a solid argument and provided multiple, authoritative sounding references to existing case law. The only problem was that whilst they looked perfect they were entirely fictitious. Safe to say the Judge was less than impressed.
The point to understand is that ChatGPT is trying to work out, statistically, what the next word should be and whilst what it concludes might look and sound right that is not a reflection, in absolute terms, that it is right. Looking right isn’t being right. A Large Language Model is not a database, it's a prediction engine. It is trying to predict what a good answer to the question being asked would look like.
This is where the abstract and critical thinking mentioned above comes into play.
Beyond hallucinations here are eight other key risks and ethical considerations to take into account when using Generative AI:
Misinformation and Deepfakes: Generative AI can be used to create realistic and deceptive content, including deepfakes, which are manipulated media that appear authentic. This raises concerns about the potential for spreading misinformation, damaging reputations, and undermining trust in media and information sources.
Intellectual Property Infringement: Generative AI models can generate content that resembles existing copyrighted material, raising concerns about intellectual property infringement. This includes the unauthorised creation of artwork, music, or written content that closely mimics the style or characteristics of original works.
Bias and Fairness: Generative AI models can unintentionally perpetuate biases present in training data. If the training data is biased, the generated content may reflect those biases, leading to unfair or discriminatory outcomes. Addressing bias and ensuring fairness in the training data and model outputs is crucial for responsible use of Generative AI.
Privacy and Data Protection: Generative AI models often require access to large datasets, which may contain sensitive or private information. Ensuring robust data protection measures and respecting privacy rights is essential to prevent unauthorised access or misuse of personal data during the training and deployment of Generative AI models.
Consent and Permission: Generating content using Generative AI may involve using data or personal information without explicit consent or proper permissions. Respecting legal and ethical requirements for obtaining consent and permissions is crucial to avoid infringing on individuals' rights and privacy.
Security and Malicious Use: Generative AI models can also be misused for malicious purposes, such as generating synthetic identities, creating deceptive content for fraud, or producing convincing phishing materials.
Psychological and Social Impact: The realistic nature of content generated by Generative AI can have psychological and social implications. For example, generated content that promotes hate speech, violence, or harmful ideologies can contribute to negative societal impacts and pose risks to public safety and well-being.
Accountability and Attribution: Generative AI raises challenges in determining accountability and attributing generated content to its creators. This can have legal and ethical implications, especially in cases where generated content is used for illegal activities, defamation, or other harmful purposes.
The overall point here is not to reduce the appeal of working with Generative AI, or to make it seem a legal minefield that might best be avoided, but to emphasise that there is a serious side to all of this that warrants serious consideration. Being aware of the situation alone goes a long way to nullify it. Again, use your abstract and critical thinking skills whenever working with these tools. And keep abreast of legislation and ‘accepted norms’; these are likely to increase and change considerably in the years ahead.
8. Task adoption - timetable to automation
I’m going to keep this section brief and rely on data put out by McKinsey in a report (The Economic Impact of Generative AI) published in June 2023.
To quote the report:
‘Based on developments in generative AI, technology performance is now expected to match median human performance and reach top quartile human performance earlier than previously estimated across a wide range of capabilities (Exhibit 6 - see below). For example, MGI previously identified 2027 as the earliest year when median human performance for natural- language understanding might be achieved in technology, but in this new analysis, the corresponding point is 2023.’
As a result of these reassessments of technology capabilities due to generative AI, the total percentage of hours that could theoretically be automated by integrating technologies that exist today has increased from about 50 percent to 60–70 percent. The technical potential curve is quite steep because of the acceleration in generative AI’s natural-language capabilities (Exhibit 7 see below).
Interestingly, the range of times between the early and late scenarios has compressed compared with the expert assessments in 2017, reflecting a greater confidence that higher levels of technological capabilities will arrive by certain time periods.
They make three other very pertinent points:
First that based on historical findings technologies take eight to 27 years from commercial availability to reach a plateau in adoption.
Second that automation adoption is likely to be faster in developed economies, where higher wages will make it economically feasible sooner.
And third that technologies could be adopted much more rapidly in an individual organisation.
Put it all together and the implications are that we are set for rapid change!
9. Impact on Professionals & Jobs
‘AI is the revenge of the working class: now it's the middle class's turn to fear for their jobs.’
Pedro Domingos, Professor of Computer Science, Washington University
This is a pithy way of putting it but it is undoubtedly true that Generative AI has really upended the consensus as to whom AI is going to have the most impact on. Because of its ability to be creative and to handle high cognitive tasks ‘Generative AI is likely to have the biggest impact on knowledge work, particularly activities involving decision making and collaboration, which previously had the lowest potential for automation.’ As stated by McKinsey but pretty much reflecting the new consensus. Because ‘its capabilities are fundamentally engineered to do cognitive tasks’.
‘Our estimate of the technical potential to automate the application of expertise jumped 34 percentage points, while the potential to automate management and develop talent increased from 16 percent in 2017 to 49 percent in 2023.
As a result, many of the work activities that involve communication, supervision, documentation, and interacting with people in general have the potential to be automated by generative AI, accelerating the transformation of work in occupations such as education and technology, for which automation potential was previously expected to emerge later’
McKinsey, in their report, are actually quite bullish for jobs and productivity. But based on the time involved with tasks being lost to Generative AI being repurposed to work on new tasks that are at least as productive as those they are substituting for.
Which strikes me as a big ask!
At least in the short term. As we know historically, whenever a new technology has displaced jobs they end up being more than replaced by new jobs that only exist because of that new technology. But we also know that there can be a very long gap between the two. In the Industrial Revolution this was known as the ‘Engels Pause’ during which living standards for the working class stagnated or declined, despite increases in productivity, and which lasted for decades.
How are knowledge workers going to create new high paying, cognitive work when ‘the machines’ can now do high end cognitive work?
The Economists Daron Acemoglu and Simon Johnson, in their recent book ‘Power & Progress’, make the point that new technology does not necessarily lead to higher living standards, but when it does it is a consequence of societal norms and beliefs that ‘will’ that to be the case. Whether AI does so or not is going to be down to whether ‘we’ are happy to go along with the structural situation where returns in technology mostly go to Capital rather than Labour.
As Mr Domingos suggests, the middle classes were not so bothered when major changes impacted the working classes, but maybe now they are in the firing line things might be different.
Either way this is too big a topic for here but two thoughts are worth bearing in mind:
First, when AI reduces the cost of writing software down to almost zero we might well see a vast increase in new software that enables us rapidly and widely to create many many new and better, faster, cheaper products and services. When the cost of intelligence trends towards zero we ‘might’ unleash all manner of new opportunities.
And secondly, with lawyers in mind, when AI enables everyone to sue everyone, everywhere, all the time, the sheer scale of new work might keep them all gainfully employed for a long time to come.
Truth is we don’t know what might come to pass, and much of the consequences will be down to actions ‘we’ take, but nevertheless it is certain that a lot of change is coming our way.
10. Impact on real estate / cities.
For this I am going to refer you to my 5 in the series set of articles about ‘Four Great Real Estate Challenges’, and my long article on ‘Cities, AI and the Metaverse? Risks, Opportunities, Actions’.
Generative AI will have an enormous impact on real estate / cities as one, and a very important one, of the forces currently at play. Between the two articles I cover most of what you need to know in some depth.
Conclusion
This is long enough for now so the 'Conclusion' is to follow:)